BPOs’ AI Pivot Playbook: Future‑Proof CX Profitability with AI
.png)
Setting the Stage: Customer Support at an Inflection Point
Precedence Research values the global BPO market at US $315 B in 2024, headed for US $840 B by 2034—but only if providers pivot to AI‑augmented delivery.
It’s fair to say that pressure is rising on every side of the outsourced-support industry. Contact volumes keep climbing even as CFOs demand leaner cost-to-serve, and customers—whether Gen Z or boomers—now expect seamless, instant answers across channels. In McKinsey’s latest benchmark, more than 80% of service leaders say they are already funding generative-AI pilots, yet only 8 percent feel their current operating model is “future-ready.” (McKinsey & Company)
Meanwhile, the stakes for business-process outsourcers have never been higher. Analysts project the global customer-experience outsourcing segment to top $525 billion by 2030, expanding at roughly 9 percent CAGR as retailers and direct-to-consumer brands look for variable-cost ways to scale service. (Exploding Topics) But growth is not guaranteed: the same forecast warns that value will accrue disproportionately to providers who embed advanced automation in their delivery stack.
That crossroads—between legacy labor-arbitrage models and AI-enabled, outcomes-based partnerships—defines the future. How BPOs respond over the next 18 months will determine whether they become strategic orchestrators of human-plus-machine support—or watch clients migrate to AI-native vendors. The following playbook outlines the choices, economics, and execution steps that will separate winners from also-rans.
AI Disruption: From Scripted Bots to Large-Language-Model Agents
The first chatbot dates back to ELIZA in the 1960s, yet most contact-center “bots” launched over the past decade were still rule-based trees that handed off anything nuanced to a human. That model is being leap-frogged at startling speed. Gartner now expects 80 percent of customer-service organizations to embed generative-AI tools in their agent workflows by 2025, up from barely 5 percent three years ago. (Webex Blog)
Three forces explain the acceleration:
- Quality leaps. Large language models (LLMs) digest unstructured voice and text, draft policy-compliant replies, and summarize multi-turn conversations in seconds. Gartner estimates those capabilities will strip US $80 billion in agent labour costs by 2026 as routine interactions shift to machines. (AIMultiple)
- Cost curves. Early adopters paid premium API fees, but the economics are tipping fast. AT&T, for example, replaced a single-vendor GPT-4 workflow with a tiered open-source stack; it retained 91 percent of the original accuracy while cutting compute spend by 65 percent and shrinking daily processing time from 15 hours to under five. (Business Insider)
- Breadth of use-cases. Beyond self-service, LLMs now draft post-call memos, recommend upsell scripts in real time, and triage sentiment across channels. Gartner projects that automated interaction handling will grow five-fold, to 10 percent of all contact-center engagements by 2026. (AIMultiple)
.png)
For BPOs built on head-count billing, these numbers are more than hype. They mark the moment when “good-enough” bot performance crosses the threshold of economic inevitability—blurring the line between technology vendor and traditional outsourcer. The next sections examine why that doesn’t spell the end of human-led service, and how BPOs can translate disruption into durable advantage.
Why BPOs Still Matter: The Human Edge in a Machine World
Headlines about 100‑percent automated contact centers obscure a simple truth: the closer an interaction gets to nuance, emotion, or high‑stakes problem‑solving, the more customers still want a human in the loop. McKinsey’s Contact‑Center Crossroads study finds that even in companies pushing hard on AI, 30‑40 percent of inbound volume today involves exceptions—refund disputes, multi‑item order failures, loyalty‑tier complaints—that machines alone cannot yet close. (McKinsey & Company)
Three complements keep BPO agents relevant:
- Empathy and trust‑repair. When a lost‑package claim becomes a social‑media flare‑up, tone and judgment matter more than handle‑time. HBR’s long‑running “collaborative‑intelligence” research shows that hybrid teams—humans supported by AI prompts—outperform either side working solo on measures of customer confidence and lifetime value. (Harvard Business Review)
- Process orchestration. E‑commerce journeys span payment gateways, 3PL trackers, and marketplace policies. Agents act as “system integrators,” stitching data silos together so AI outputs translate into concrete actions—refunds issued, reships booked, courier escalations triggered.
- Relationship management. Brands increasingly treat support touch-points as micro‑moments for upsell or retention. Skilled agents convert an apology into an exchange, a warranty claim into a subscription renewal—tasks that require persuasion techniques LLMs have not yet mastered within compliance guardrails.
In short, automation may shrink repetitive tickets, but it elevates the strategic value of the human layer. For BPOs, the opportunity is to redeploy capacity from rote Q&A to these higher‑order roles, capturing margin upside rather than ceding volume to AI‑native entrants.
Strategic Choices Ahead: Compete, Pivot, or Partner
Generative-AI adoption is no longer a tech experiment; it is a board-level fork in the road. McKinsey’s three-horizon model for contact-center AI shows that only 12 percent of early adopters have scaled beyond proof-of-concept, while the rest are “stuck in pilot purgatory,” weighed down by integration debt and unclear ROI. (McKinsey & Company)
Against that backdrop, BPO leaders face three—and only three—moves:
Compete (go it alone)
A handful of tier-one outsourcers are investing in proprietary LLM stacks, hoping to protect time-and-materials revenue. Yet a16z warns of a fundamental business-model mismatch: labor-based margins vanish when AI handles the work, forcing incumbents to cannibalize their own billing to win. (Andreessen Horowitz)
Pivot (become AI-first)
Some mid-market players are re-tooling entirely—shifting from seat-count pricing to outcome-based contracts, hiring data engineers, and packaging advisory plus systems-integration services. Parloa’s analysis suggests those that succeed unlock three new revenue streams—advisory, integration, and SaaS—worth up to 30 percent incremental margin. (Parloa)
Partner (embed best-in-class AI)
The fastest path is to integrate off-the-shelf automation from specialist vendors, then layer human orchestration and relationship management on top. Early partnerships cut time-to-market from quarters to weeks and let BPOs monetize AI without the cap-ex or research burden—an approach we will unpack in Section 10.
Choosing among these plays is not merely a technology decision; it defines cost structure, sales narrative, and client perception for the next decade. The window to decide is narrowing as retailers benchmark suppliers on AI capability in RFPs.
Partnership Playbook: Embedding AI Within the BPO Offering
For outsourcers that lack the balance sheet—or the appetite—to build their own generative-AI stack, the fastest path to relevance is partnership. Good alliances swap complementary assets: the AI vendor supplies pre-trained models, connectors to the likes of Shopify or Zendesk, and a usage-based commercial model; the BPO brings deep process knowledge, multilingual agents, and account control. The result is an integrated service that can be priced on business outcomes rather than labour hours.
In practice, a partnership unfolds in three layers. First comes technology embedding: the BPO hosts the AI inside its existing telephony or help-desk workflow so escalations, tags, and CSAT scores flow into one pane of glass. Invoca’s 2025 analysis shows that when AI handles quality-assurance scoring and intelligent routing natively, contact centers cut agent re-work by up to 25 percent and slice average handle time by several minutes without touching their CRM stack. (invoca.com)
Next is commercial packaging. Leading providers anchor client pitches around a blended automation metric—say, “40% of tickets resolved by machines within 60 seconds”—and peg fees to that outcome. Because the AI partner typically charges only for fully resolved interactions, margin expansion accrues to the BPO, not the software vendor.
Finally, there is joint go-to-market learning. A North-American outsourcer that embedded an e-commerce-focused AI agent in 2023 reached 50 percent full automation for its top retail clients within months, slashing first-response time from seven hours to one and freeing nine staff-days per month at a single brand. (yuma.ai) Crucially, the outsourcer retained ownership of the relationship while the AI provider handled model tuning and roadmap updates—illustrating how each party stays in its lane yet shares upside.
When executed this way, partnership is not a defensive hedge; it is a route to new revenue lines (integration services, data analytics, conversational-commerce upsells) and to a valuation multiple that looks a lot more SaaS than staffing.
Economics of Automation: Margin Expansion and New Revenue Lines
Generative-AI is rewriting the P&L of customer-support outsourcing. On the cost side, fully automated resolutions can be delivered for pennies compared with the blended $2 to $4 per chat or email that BPOs typically bill today. Deloitte’s 2024 enterprise survey reports that 74 percent of adopters already see positive ROI, and one in five posts gains above 30 percent—figures reached within the first 12 months of deployment, not years.
Case data reinforce those averages. When AT&T rebuilt its call-analytics stack around open-source LLMs, it preserved more than 90 percent of classification accuracy while cutting compute spend by 65 percent and trimming analysis cycles from hours to minutes. Even modest automation rates—say 25 percent of tickets—translate into double-digit margin expansion for a BPO once seat-based overhead is removed from the workload.
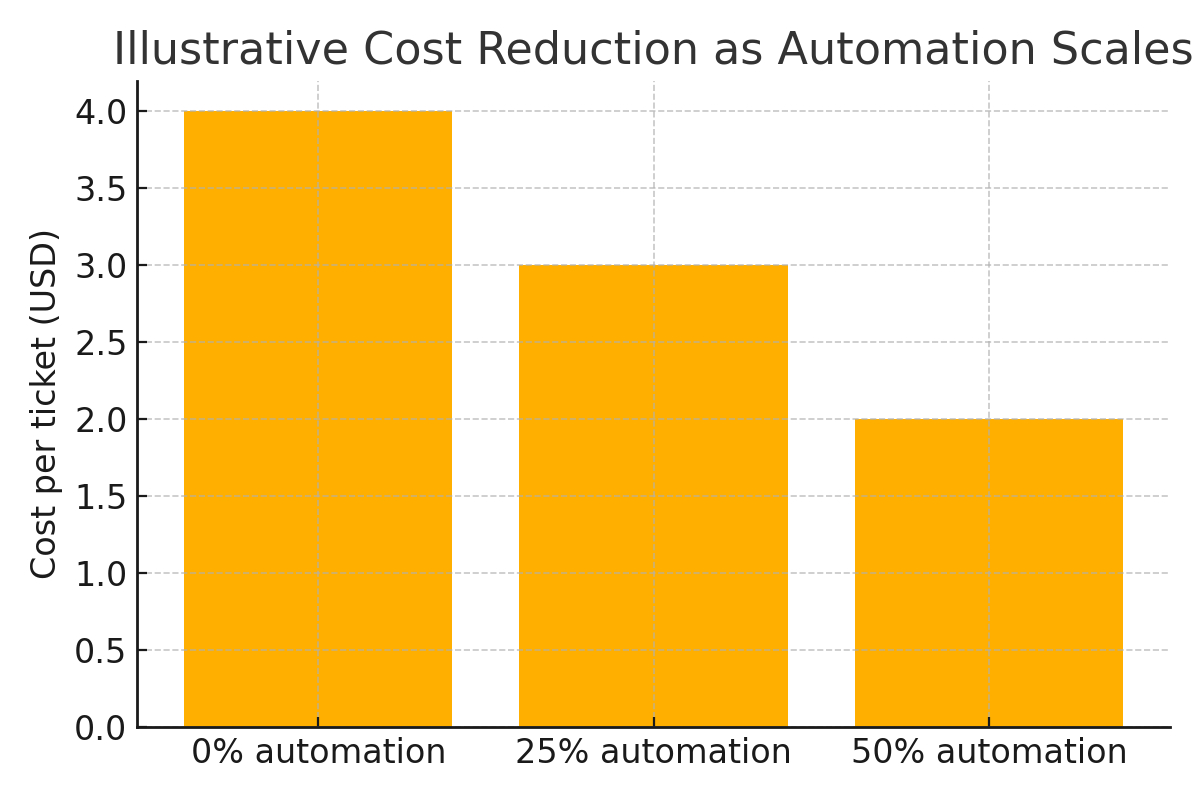
Yet the upside is not limited to cost. McKinsey finds that AI-powered intent prediction lifts cross-sell conversion by three to five percentage points; in subscription commerce, that equates to $15 to $25 of incremental lifetime value per retained customer. High performers are bundling those gains into outcome-priced contracts: a retailer pays a bonus for reduced churn or higher basket size, aligning incentives with the BPO’s AI layer rather than agent handle time.
The net result is a new economic equation: lower unit costs free capacity for specialist human work, while AI-driven insights open fresh revenue pools—shifting the provider’s story from “cheaper labour” to “growth enabler.”
Safeguards & Governance: Quality, Compliance, and Trust
Speed without safeguards is a reputational hazard. BPOs that embed generative AI inherit liability for data leaks, biased responses, or hallucinated promises—risks that regulators are moving to codify. The NIST AI Risk-Management Framework 1.0 calls for documented model provenance, continuous monitoring, and human-override controls at every stage of the AI lifecycle, from design to decommissioning.
Europe is going further: the EU AI Act, slated to begin phased enforcement in 2025, imposes strict transparency and accuracy thresholds on “high-risk” customer-interaction systems and fines of up to 7 percent of global turnover for violations. Outsourcers that serve EU consumers—or even process their data offshore—must therefore prove they can detect toxic content, audit prompts, and maintain clear escalation paths.
Leading providers embed a triad of guardrails:
- Model governance—version-controlled prompts, bias testing, and alignment checks baked into release pipelines.
- Operational controls—service-level targets (latency, resolution accuracy) monitored in near real time, with automated fall-back to human agents if thresholds slip.
- Contractual transparency—shared dashboards that let brand clients see precisely when a machine versus a person handled an interaction and how the AI arrived at its answer.
Guardrails may feel like overhead, but they are fast becoming table stakes for winning RFPs—turning “responsible AI” from a compliance tick-box into a competitive differentiator.
Roadmap to 2026: A Phased Approach to AI Adoption
Generative-AI maturity does not happen in a single platform launch; it unfolds through deliberate capability building. Gartner’s adoption curve suggests that providers who sequence investments over 24-to-30 months realize twice the automation lift of “big-bang” roll-outs.
Phase 1 — Enable (0-6 months). BPOs start by wiring LLM copilots into existing workflows: summarizing tickets, suggesting macros, flagging churn risk. The goal is agent productivity—often a 15-to-30 percent handle-time drop—without client-visible change.
Phase 2 — Scale (6-18 months). With data pipelines hardened, providers expose AI directly to customers for low-risk intents (order-status, FAQs) and switch commercial terms to outcome-based pricing. Continuous-learning loops refine accuracy, pushing automation past the 40-percent mark.
Phase 3 — Reinvent (18-30 months). The operating model flips: humans supervise exception handling while AI owns the long tail of routine work. New revenue engines—speech-analytics insights, personalized upsell triggers—emerge as core products, not add-ons.
Harvard Business Review notes that organizations making this leap share a leadership pattern: cross-functional squads that blend data science, CX design, and frontline supervisors under a single P&L persona—an approach that accelerates experimentation and de-risks change fatigue.
For BPOs, mapping initiatives to these milestones clarifies budgeting, talent needs, and client messaging—turning an abstract technology trend into a time-boxed execution agenda.
The Cost of Inertia: Scenarios for Late Movers
Staying on the sidelines now carries a clear price tag. Forrester’s 2025 outlook warns that the revenue pool is about to be redistributed, not expanded: “smart BPOs will shift away from the classic FTE model and adopt outcome-based pricing,” it notes, leaving laggards to defend a shrinking labour-hour pie. (LinkedIn)
A companion forecast underlines the consequence—only those willing to “kick things into high gear” will out-grow a year of CX mediocrity. (Forrester)
What does that look like in practice? Consider two plausible futures for a mid-market outsourcer serving global e-commerce brands:
Scenario A—Early Adopter
The provider embeds generative-AI during 2025, reaches 40 percent automation by Q4 2026, and pivots contracts to resolution-based pricing. Net margin lifts by 8–12 points, according to Deloitte ROI ranges, freeing capital to hire multilingual client-success managers. Automation analytics reveal new upsell moments that add two percentage points to client revenue—a gain the provider shares in a bonus pool.
Scenario B—Late Mover
The same firm delays investment, holding to seat-based billing while clients benchmark against AI-native vendors. By 2027 it faces double jeopardy: a 15-percent rate cut in renewal negotiations and mounting talent attrition as agents question the career path. Computer Weekly’s legal-practice analysis predicts “material staff attrition at certain levels” for providers that fail to redeploy workers up the value chain, alongside new regulatory costs to audit legacy processes retroactively. (Computer Weekly)
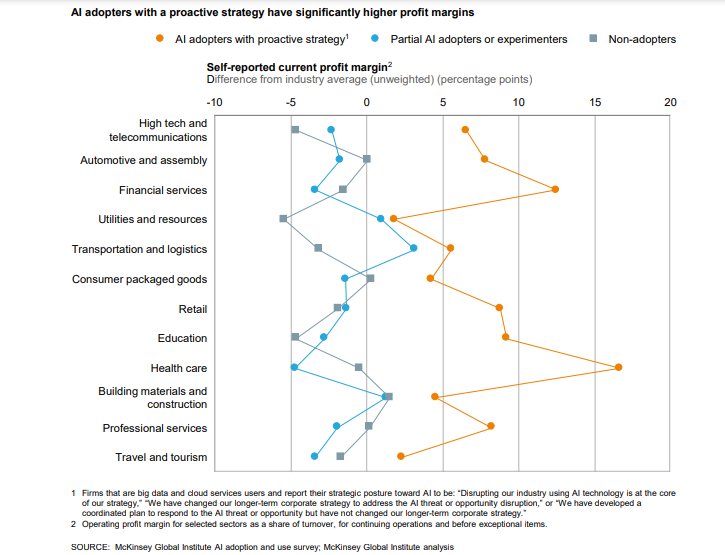
The delta between the two paths compounds quickly: in a typical 500-seat operation, that margin swing equals several million dollars of annual EBITDA—enough to fund continued AI reinvestment or, conversely, to trigger a valuation write-down that makes the business a takeover target. Put differently, choosing to wait is itself a strategic decision—one that quietly mortgages future optionality.
Case Spotlight: Omnie + Yuma—A Blueprint for Co-Selling Automation
When North-American outsourcer Omnie confronted a surge of multilingual, round-the-clock queries from its fast-growing e-commerce roster, leadership chose not to build an AI stack from scratch. Instead, the firm embedded a specialist e-commerce automation platform (Yuma) directly into its help-desk workflow. The two companies agreed on a simple commercial principle: the AI vendor charges only for fully automated resolutions, while Omnie retains the client relationship and layers human value on top.
Execution. The integration launched in April 2023. Within weeks, AI agents were handling routine “where’s-my-order” and return-policy tickets across time zones and languages. Deep Shopify connectors gave the system real-time order data; brand-persona controls let Omnie match each merchant’s tone. By month six, Omnie had rolled the model out to a dozen online retailers.
Results. Top merchants now achieve ≈ 50 percent full automation, cutting first-response time from seven hours to about one and saving one client the equivalent of nine staff-days in a single month. CSAT scores on AI-resolved interactions register between 4.5 and 5 stars, occasionally surpassing human-handled threads.
Transferable lessons for other BPOs
- A reseller arrangement can be inked in weeks when pricing aligns to outcomes, not licences.
- Start with low-risk intents to build confidence, then expand to returns, warranty, and proactive upsell triggers.
- Maintain a joint roadmap with quarterly “learning reviews”; Omnie credits rapid feature roll-outs—handled by the AI partner’s engineering team—for its ability to ramp new brands quickly.
The takeaway is not that every outsourcer must find the same vendor; rather, it is that structured partnership can deliver double-digit automation, higher margins, and better customer experience—without sacrificing the human expertise that keeps complex e-commerce shoppers loyal.
Install Yuma for:
