How accurate is AI in customer support?
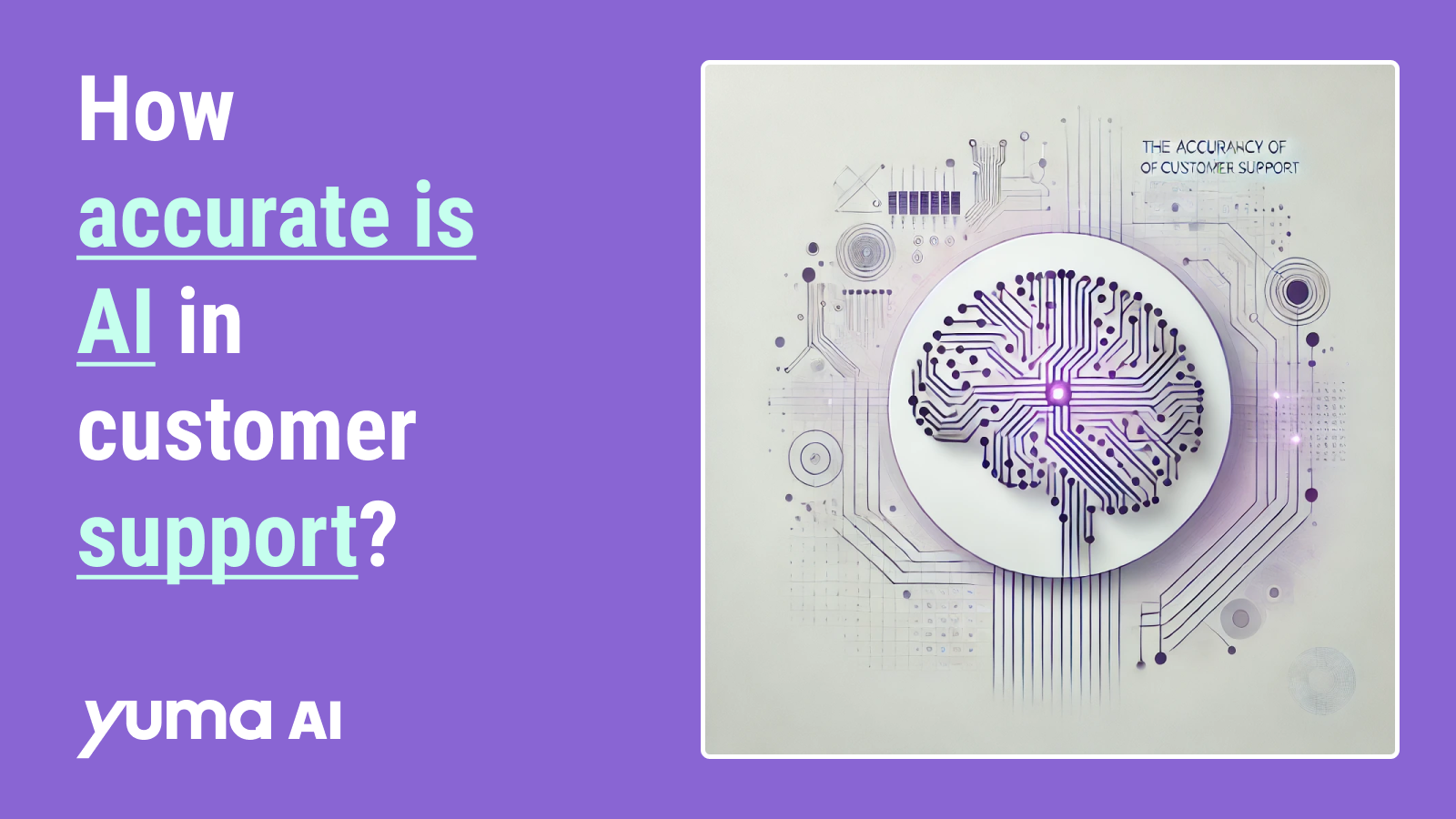
Answer: AI tech for customer support is pretty accurate after the advent of Gen AI. In our cases we fully automate 50% of all support tickets for our top customers. That doesn’t mean AI is 100% error free. Hence, the rest of AI accuracy depends on the developers of the AI tool and how much work they have done around core AI to make it accurate for support!
Decision-makers across industries are rapidly embracing artificial intelligence (AI). According to Forbes Advisor, 64% of business owners believe AI will improve their customer relationships.
Customer service professionals echo similar sentiments. According to HubSpot, 79% view AI and automation tools as essential to their overall customer experience (CX) strategy, and 78% say these tools help them spend more time on the most important parts of their role.
With buy-in from both executives and customer service representatives, it’s no surprise that AI is making significant waves in e-commerce. Companies that successfully integrate AI into their CX processes often see rapid ROI and significant growth in customer satisfaction.
However, AI isn’t unimpeachable — from AI hallucinations to contextually incorrect outputs, it has shortcomings that require programming. Partnering with an AI vendor that has robust guardrails in place is crucial to ensure accuracy, reliability, and alignment with business objectives.
In this blog, we’ll review the state of AI in CX and detail what to look for in an AI vendor.
Capabilities and limitations of AI
AI technology has made remarkable strides in recent years. Even so, there remain limitations that make human oversight essential.
Advancements in AI
Today’s AI can process vast amounts of data in real-time, identify patterns, and deliver personalized responses that closely mimic human interactions. Several recent advancements have made this possible:
- Machine learning algorithms: These algorithms enable AI to learn from data and improve over time, enhancing its performance and adaptability. Ultimately, machine learning allows AI systems to better understand patterns and make accurate predictions.
- Advanced neural networks: These models make decisions in a manner similar to the human brain, using processes that resemble the way biological neurons work to understand and spot patterns. Neural networks have significantly advanced the field of AI, particularly in image and speech recognition.
- Natural language processing: Natural language processing allows AI to understand and generate human-like text responses, making interactions more natural and intuitive. This is particularly important in customer service, where clear and seamless communication is essential.
- Deep learning: A subset of machine learning, deep learning utilizes multi-layered neural networks to analyze data. This approach has been particularly successful in image and speech recognition, as well as natural language processing, due to its ability to model complex patterns in large datasets.
- Reinforcement learning: Reinforcement learning is an area of machine learning where AI systems learn by interacting with their environment and receiving feedback in the form of rewards or penalties. This is particularly effective for tasks that require a sequence of actions to achieve a desired goal, such as robotics and game playing.
Limitations of AI
Despite these technological leaps, AI nonetheless requires human oversight to perform its best. It has certain limitations, such as:
- Inability to fully comprehend complex queries: While AI excels at handling straightforward, repetitive tasks, it sometimes struggles to manage requests that involve subtle, ambiguous, and context-specific nuances. This limitation underscores the need for a hybrid approach, where AI handles routine queries and human agents address more complex issues.
- Inaccurate information: According to the HubSpot study, 55% of customer service specialists believe generative AI tools like ChatGPT, Claude, and Bard sometimes offer incorrect information, and only 42% think they’d be able to spot it when it happens.
AI applications and operational challenges in CX
Technological advancements have caught the attention of business leaders. According to Forbes Advisor, 64% of companies believe AI will increase their overall productivity, demonstrating growing confidence in its potential to overhaul operations and customer interactions.
Applications
The HubSpot survey identified several ways e-commerce businesses are using AI and automation tools to enhance their CX. Notably:
- 41% use AI agents to respond to customer service requests
- 41% use generative AI to write responses to customer queries
- 38% use AI routing tools to send requests to the right agent
- 37% use AI to prioritize requests by urgency
- 37% use AI to collect and analyze customer feedback
Operational challenges
Despite its widespread adoption, AI is not without inherent challenges. In addition to questions about accuracy, concerns linger about:
- Preventing AI hallucinations: Incorrect or misleading results generated by AI, known as AI hallucinations, are a fact of life in even the very best language learning models. According to GitHub, the top-rated AI model (GPT-4 Turbo) is factually incorrect 2.5% of the time, a small yet significant amount. Other models answer incorrectly at higher percentages. AI hallucinations have several causes — biased data, insufficient training data, and incorrect assumptions, among others — but no matter the origin, AI vendors must build guardrails around it to avoid misleading their customers.
- Managing ambiguous requests: AI systems rely on predefined parameters and datasets, making it difficult to interpret questions that are vague or unclear. The AI can go off topic and provide irrelevant responses when a request is too ambiguous or has human error.
- Ensuring contextual understanding: AI lacks the deep contextual awareness of human agents, which can result in technically correct but contextually inappropriate replies.
- Gaining customer buy-in: While business leaders are largely embracing AI, consumers aren’t quite all-in. According to Forbes Advisor, 75% of consumers are concerned about misinformation from AI, and 58% have concerns about AI agents being used to answer questions.
The importance of guardrails in AI
Although research and development efforts aimed at addressing AI hallucinations, limitations in contextual understanding, sentiment analysis, and AI training methodologies are ongoing, AI isn’t perfect — not yet, at least. A majority of decision-makers and customer service representatives see the potential for AI to enhance productivity and improve customer relationships, but ensuring a smooth rollout is a whole other animal.
E-commerce businesses can implement AI in their CX and be reasonably confident they’ll respond to customers faster. And that matters — StatSocial confirms that 50% of customers are less likely to spend money with a business that takes too long to respond. But, given that even the best AI models are prone to inaccuracies, how do they know they’re answering correctly and within the right context?
Our approach
This brings us back to the theme of guardrails. Yuma AI has a specific and intentional approach to ensuring timely and accurate responses from its AI agents.
- Specific intents: Our AI agents are triggered only on very specific intents, preventing a single AI from attempting to handle every type of message. This careful linking of messages to appropriate automations leads to greater accuracy.
- Restricted automations: Each automation focuses on a set of allowed topics. If the AI encounters an issue outside of its scope, it escalates the case to a human agent, ensuring no incorrect information is relayed.
- High-quality models: We use the best and most advanced models available, prioritizing top-notch performance without compromising on quality.
- Policy checks: Every message generated by our AI undergoes strict policy checks, akin to having a manager review each message against a set of rules. This facilitates consistency and adherence to brand standards.
- Quality control: Built-in quality control checks are run on each message, ensuring outputs are verified through a long chain of thoughts and validations. This additional layer of scrutiny helps maintain a high level of accuracy and reliability.
The future of e-commerce with Yuma AI
AI is transforming customer service, offering unprecedented opportunities for efficiency, scalability, and improved customer satisfaction. What’s more, it’s not just decision-makers who see the opportunity — according to HubSpot, 71% of customer service professionals say automation helps them spend more time on the parts of their role they enjoy most.
The implementation of AI in CX requires a balanced approach and sufficient safeguards. You’ll want to partner with a company that has a track record of success, emphasizes security, and has protections in place to ensure the AI is accurate.
Yuma AI exemplifies this balanced approach. By leveraging specific intents, restricted automations, high-quality models, stringent policy checks, and comprehensive quality control, we deliver reliable and accurate AI-driven customer service solutions. Plus, our technology fully automates up to 50% of CX tasks, ranging from order and subscription management to shipping and post-sale troubleshooting.
Ready to take your e-commerce CX to the next level? Contact us today.
Install Yuma for:
