Why CSAT Fails in Evaluating AI-Powered Customer Support Performance
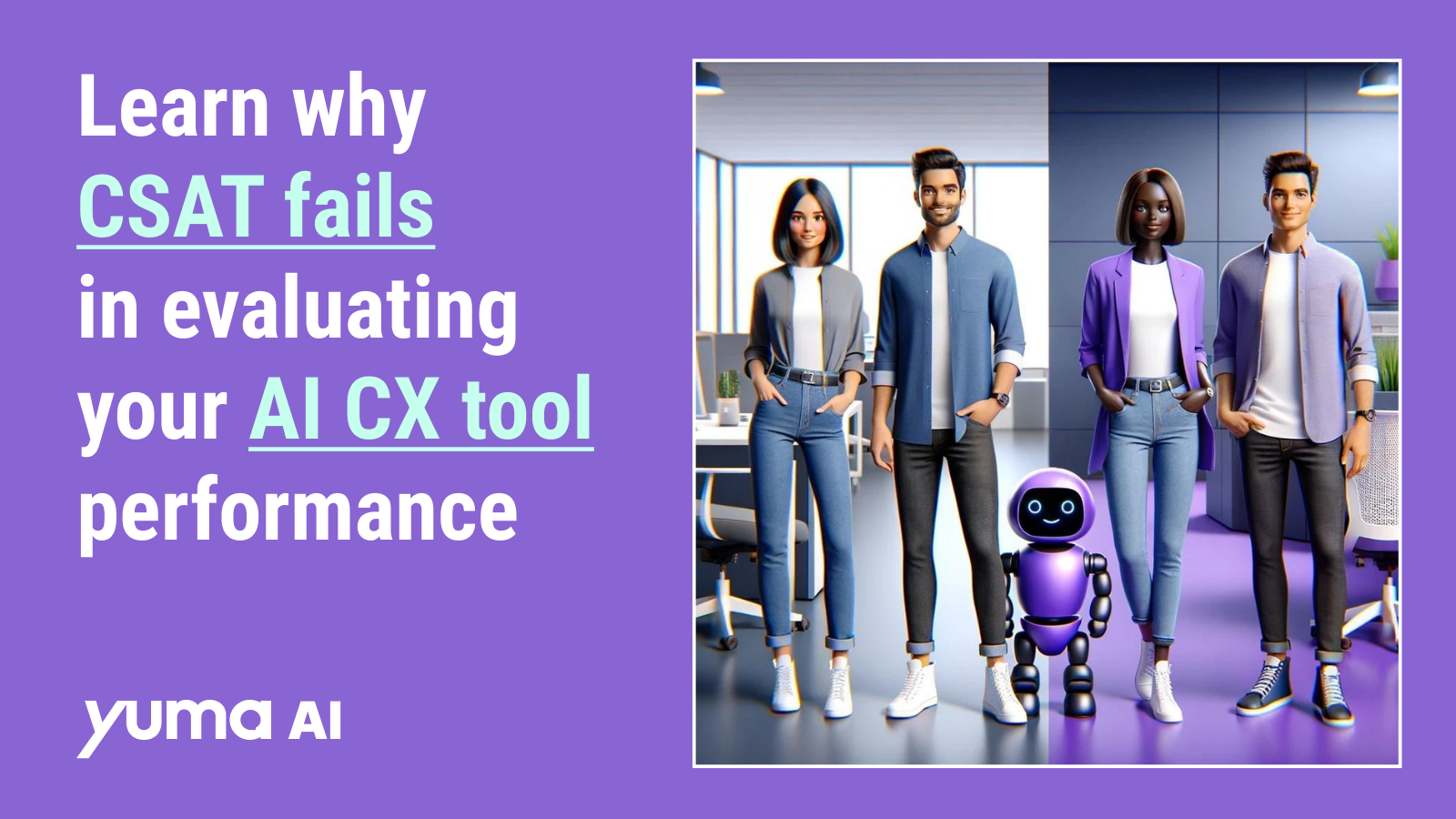
Yes, CSAT is not fit to evaluate your AI performance. It was potentially okay without AI, but with AI in the mix, it's now an outdated tool. Let me explain why below.
Some background on CSAT first
Despite its limitations, CSAT is widely used in the customer service industry as a key metric to gauge the quality of the support department. Despite its imperfections, CSAT's simplicity and widespread adoption have made it a universally recognized standard.
CSAT is a score provided by a customer to rate the quality of support received, usually measured on a 1-5 scale in the e-commerce industry.
However, CSAT does have known biases, primarily the Response Bias and the Temporal Bias. Often, only dissatisfied customers may take the time to grade your service. Additionally, the score is typically taken shortly after an interaction and may not reflect the customer's entire journey. Adding to this the fact that CSAT is highly dependent on the business model and the products being sold. CSAT can vary widely between merchants, not necessarily always due to the quality of their support team.
With that in mind and despite those flaws, CSAT can still be considered a good general indicator to monitor the health of your support organization.
AI to the rescue
The introduction of AI is a significant shift, offering numerous benefits to your customers.
To state the obvious, AI ensures 24/7 support, faster response times, higher overall ticket quality thanks to a shared knowledge base, and streamlined centralized procedures.
While AI is a major boost to your support organization, it's crucial not to rely solely on CSAT to gauge its efficiency. Even though some AI tools out there are touting their AI CSAT, here's why you shouldn’t take those scores at face value.
AI vs Humans
By default, your AI will begin by handling the simplest cases and answering those quickly, often resulting in higher CSAT scores. This is because it's relatively easy for any good AI to achieve good CSAT, especially as they tend to handle cases that align with customer expectations, such as avoiding denying refunds. Your AI having a good CSAT is basically a requirement and it’s easy to achieve.
However, what happens next? Your human team is left with the more complex cases, the tickets that might go against the customer's wishes, or issues dealing with real problems, such as a lost package, which are more likely to result in lower CSAT scores.
Consequently, when you split your CSAT scores into human vs AI, you're comparing two very different datasets. This means your comparison is completely biased. Finally, as your AI scales, its CSAT score remains high and steady, while your human CSAT score may continue to decrease as they are left with the more arduous cases.
This is unfair to your human team and might give you a misleadingly positive impression of your AI. The AI is simply handling the easier tasks and might not actually be doing the hard work.
If you still want to use CSAT, at least try comparing tickets with similar intents. This should provide a more accurate picture of how your AI is truly performing (filter by tag or ticket fields for example). Also, it goes without saying, but pick an AI tool that can truly automate your support. You want autonomous AI agents that can fetch information from external services as well as take actions in those, ie: truly automating, not just answering simple Q&A about your business. This means dealing with L2 and L3 tickets, not just L1.
A New Industry-Wide Scoring with AI in Mind?
Clearly, as every merchant is adopting AI to improve the quality and efficiency of their support, we need to rethink our approach to tracking the quality of each interaction. This likely involves creating a new score ready for the AI age, one that isn't biased by policy enforcement, speed, or mistakes beyond the control of the support agents.
At Yuma, we're developing an alternative scoring system that we plan to release this coming June. Our goal is to create a system that's fair to humans, and that can assess both the quality of overall interactions and adherence to policies. If you have any ideas for what we should include in this new scoring system, please share your insights! What would be the perfect scoring mechanism for you? Can a single score actually be perfect?
To conclude, while CSAT is still a reasonably good proxy overall, please avoid using it to distinguish between Humans and AI. Or if you still do, do it while being fully aware of all the biases in that split :)
Install Yuma for:
